Alexander Waibel
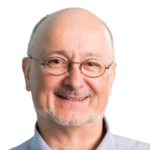
Prof. Alexander Waibel
Carnegie Mellon University, USA
Karlsruhe Institute of Technology, Germany
Alexander Waibel is Professor of Computer Science at Carnegie Mellon University (USA) and at the Karlsruhe Institute of Technology (Germany). He is director of the International Center for Advanced Communication Technologies. Waibel is known for his work on AI, Machine Learning, Multimodal Interfaces and Speech Translation Systems. He proposed early Neural Network based Speech and Language systems, including in 1987 the TDNN, the first shift-invariant (“Convolutional”) Neural Network, and early Neural Speech and Language systems. Based on advances in ML, he and his team developed early (’93-’98) multimodal interfaces including the first emotion recognizer, face tracker, lipreader, error repair system, a meeting browser, support for smart rooms and human-robot collaboration. Waibel pioneered many cross-lingual communication systems that now overcome language barriers via speech and image interpretation: first consecutive (1992) and simultaneous (2005) speech translation systems, road sign translator, heads-up display translation goggles, face/lip and EMG translators. Waibel founded & co-founded more than 10 companies and various non-profit services to transition results from academic work to practical deployment. This included “Jibbigo LLC” (2009), the first speech translator on a phone (acquired by Facebook 2013), “M*Modal” medical transcription and reporting (acquired by Medquist and 3M), “Kites” interpreting services for subtitling and video conferencing (acquired by Zoom in 2021), “Lecture Translator”, the first automatic simultaneous translation service (2012) at Universities and European Parliament, and STS services for medical missions/disaster relief.
Waibel published ~1,000 articles, books, and patents. He is a member of the National Academy of Sciences of Germany, Life-Fellow of IEEE, Fellow of ISCA, Fellow of the Explorers Club, and Research Fellow at Zoom. Waibel received many awards, including the IEEE Flanagan award, the ICMI sustained achievement award, the Meta prize, the A. Zampolli award, and the Alcatel-SEL award. He received his BS from MIT, and MS and PhD degrees from CMU.
From Dictionaries to Language Transparence
Breaking down language barriers has been a dream of centuries. Seemingly unsolvable, we are now lucky to live in the one generation that makes global communication a common reality. Such global transformation has become possible through revolutionary advances in AI, language and speech processing. Indeed, the challenges of processing spoken language have required, caused, guided and motivated the most impactful advances in AI. During a time of knowledge-based speech and language processing, we became convinced that only data-driven machine learning can reasonably be expected to handle the complexities, the uncertainty, and variability of communication, and that only latent learned representations would be able to abstract and fuse new and complementary knowledge.
It turned out to work beyond our wildest expectations. Starting with small shift-invariant neural networks (TDNN’s) for phonemes, we would eventually scale neural systems to massive speech and interpretations systems. From small vocabulary recognition, we could advance to simultaneous interpretation, summarization, interactive dialog, multimodal systems and now automatic lip-synchronous dubbing.
In the first part of my talk, I will revisit some of our earliest prototypes, demonstrators, and their transition into start-up companies and products in the real world. I will highlight the research advances that took us there from brittle early attempts to human parity on popular performance benchmarks and the lessons learned. In the second part I will discuss current research and a roadmap for the future: despite spectacular advances, the dream of barrier free communication between all the peoples on the planet has not yet been reached. What is the missing science and how can we approach the remaining challenges? It will require a more adaptive, incremental and interactive approach to learning rather than repeated batch training of ever larger systems. We call it “Organic Machine Learning”.
The talk will include demos and examples of SOTA speech translation and dubbing systems