Olgica Milenkovic
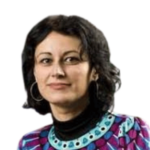
Prof. Olgica Milenkovic
10:15 – 11:15 (IST) | April 10, 2025 (Thursday)
Department of Electrical and Computer Engineering, University of Illinois, Urbana-Champaign
Olgica Milenkovic is the Franklin W. Woeltge professor of Electrical and Computer Engineering at the University of Illinois, Urbana-Champaign (UIUC), and Research Professor at the Coordinated Science Laboratory. She obtained her MS degree in mathematics in 2001 and PhD in electrical engineering in 2002 both from the University of Michigan, Ann Arbor. Prof. Milenkovic heads a group focused on addressing unique interdisciplinary research challenges spanning the areas of algorithm design and computing, bioinformatics, coding theory, machine learning and signal processing. Her scholarly contributions have been recognized by multiple awards, including the NSF Faculty Early Career Development (CAREER) Award, the DARPA Young Faculty Award, the Dean’s Excellence in Research Award, the University of Michigan ECE Distinguished Educator Award and several best paper awards. In 2013, she became a UIUC Center for Advanced Study Associate and Willett Scholar while in 2015 she served as Distinguished Lecturer of the Information Theory Society. In 2018 she became an IEEE Fellow. Prof Milenkovic has also served as Associate Editor of the IEEE Transactions on Communications, the IEEE Transactions on Signal Processing, the IEEE Transactions on Information Theory, the IEEE Transactions on Molecular, Biological and Mule-Scale Communications and the Transactions on Machine Learning Research. In 2009 and 2020 she assumed the role of Guest Editor-in-Chief of the IEEE Transactions on Information Theory on Molecular Biology and Neuroscience and the Memorial Tribute to V. I. Levenshtein.
Learning and Signal Processing Methods for Emerging Multiomics Data
Abstract: Multiomics data is a complex collection of multimodal measurements Of genomic, proteomic, transcriptomic and other -omic features which lies at the heart of modern medical and biological sciences. Multiomics data offers unprecedented insights into cellular processes and intracellular interactions, and significantly improves early disease diagnostics, personalized treatment and other intervention methods. Notable examples include chromatin interaction data, which captures physical contact maps Of folded DNA strands and corresponding gene activities, and spatial transcriptomics data, which provides information about gene activity levels at different spatial locations in a tissue. Because of the unique format and properties of multiomics datasets, many relevant learning and signal processing methods appropriate for prediction and inference are lacking or underdeveloped. We will review relevant -omics data modalities and describe a collection of new interpretable machine learning methods that are tailor-made for the data at hand and which allow us to reverse engineer gene regulation and interaction graphs, elucidate cell functionalities and infer important components of cellular communication mechanisms.